No use crying over...: a teaching strategy to tame ChatGPT into being your students’ and your best friend.

Brief Abstract
Our objective is to provide high-level understanding of LLMs, such as ChatGPT, to make instructors comfortable enough to start using these tools with students. We help instructors identify the assignments that had already been disrupted, and show how to use systematic approaches to remake them for this new reality.
Presenters
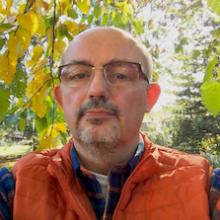
Extended Abstract
Key objectives:
- to provide high-level, basic understanding of how LLMs operate
- to place LLMs in the context of culture to understand the magnitude and weight of this advance
- to focus attention on ethical, epistemological, and methodological issues that each potential solution must consider
- to help instructors develop a strategy to identify the assignments in their existing courses had already been disrupted (and should not be re-used "as is")
- to offer instructors a simple and flexible but radical toolkit (available under CC BY-NC-SA license) that will allow them to transform their existing disrupted assignments into formats that can coexist with, and even benefit from a structured LLMs use in the new post ChatGPT reality.
Interactivity / Participant Engagement (note: wile not required for most participation, participants will be encouraged to have an active (free) Chat GPT account):
- several polls are planned during the presentation:
- know-the-context: a survey of instructors and instructional support staff on their pre-workshop approaches to identifying disruption in existing assignments;
- pre-view: asking the audience to guess the main types of AI hallucinations and then grouping their answers and discussing in reference to established / usual classification
- applying the knowledge: classifying several different examples of hallucinations
---
Summary
The email landed in our academic technology team mailbox, and it had been written with an obvious sense of urgency and apprehension: "I feel really naive," confessed one of our stellar instructors, an experienced NP (nurse practitioner) who treats dozens of patients in her clinical practice. “I just realized - she wrote - that my (graduate) students have been using Chat GPT. Some of them are pretty obvious. I do have them use Turnitin for the final paper, but I'm not sure if it checks for AI..."
First, let’s point out that by being able to detect the use of LLM at all, our instructor had already proven to be far from naive, and in fact well ahead of the crowd, but her email was a harbinger of a looming crisis that calls for a radical reconceptualization of many aspects of how we teach writing - and not only - in college courses.
The availability of LLM-based tools that use conversational, everyday language Q&A format to communicate, rather than computer code or complex specialized syntax, has made them immediately and widely accessible and unleashed them onto the world, ready or not.
GPT in Chat GPT (hence CG), stands for "Generative Pre-trained Transformer." And as GG itself explains (we asked for a sixth-grader level explanation), “it's a computer program that can do a lot of things with words, like writing stories or translating. The 'generative' part means that it can create new text that looks similar to the text it has seen before: a new story that sounds like it was written by a human. The 'pre-trained' means that it had learned a lot about language before it even starts working on a specific question, by reading a lot of text, so that it can understand how words are used and how sentences are structured. Finally, the 'transformer' part describes the way it's designed. It's like a big puzzle that can put together words in the right order to make sense. It's really good at understanding long sentences and paragraphs of text.“
In the first part of this presentation we provide a general, high-level overview of what Large Language Models (LLMs) are, and how they work. We then consider LLMs in the context of three key concepts related to technological change and its societal impact, that allow us to understand the magnitude of this technological advance: "disruptive innovation" (Christensen), "exponential technological leap" (Moore and Kurzweil), and "paradigm shift" (Kuhn). We evaluate the potential of the technology to disrupt common, well-established educational teaching and learning practices (a lecture, a research paper, preparing for an exam, and so on).
We then introduce, explain, and operationalize (show how to implement in practice) the DI-AMP (disruptive impact analysis and mitigation process) that we developed specifically to address this formidable challenge. DI-AMP is a workflow (you can think of it as a step-by-step toolkit) that guides instructors through a process of identifying the assignments in their course(s) that have been disrupted by this technology: many instructors are not aware that the disruption had already taken place, and that the papers they are painstakingly correcting were not entirely written by a human. Once the disruption points are identified, we offer specific procedures and alternatives instructors can use to update the assignments to be a better fit for the CG world in which we now all exist.
Our disruptive impact analysis and mitigation process (DI-AMP) considers the LLM challenge holistically, taking into account three aspects of using LLMs in college courses that are helpful in identifying and understanding the challenge, defining its boundaries, and developing viable, functionally sound solutions. The three aspects we address are: ethical, epistemological, and methodological.
Ethical use considerations include creating well-reasoned guidelines for using LLM in course assignments (acknowledging, citing, limits on use, including full text of the base/source-chat sequence as an appendix, to mention just a few). We provide ready-to-use how-to instruction examples )copy-and-paste ready), course and assignment guideline sheets, and assignment checklists (all available under CC BY-NC-SA license).
Epistemological questions include understanding the importance of the balance between what is already known and included in the prompt (question posed to LLM) and what output is produced, a challenge that is succinctly, if crudely expressed by the old acronym GIGA (garbage in, garbage out). We look at how to build conceptual and procedural knowledge to ensure best results, including prompting (aka prompt engineering).
Finally, in focusing on the methodological aspect we explore common risks of relying on LLM output (types of common errors aka hallucinations and identifying them, spotting biases that may reflect the training dataset), and ways to bring all three aspects (ethical, epistemological and methodological) bear on actual practices and strategies.
At this stage we also recognize that DI-AMP is a transitional tool, a stop-gap measure created to let instructors adapt existing assignments to the new reality, before assignments that tacitly and automatically assume that LLMs are available and are being used become routine. DI-AMP is also a stop-gap measure for students that re-designs assignment to combine specific academic field subject matter with LLM skill development as simultaneous and parallel processes, until students are able to develop the basic LLM prompting (aka prompt engineering) skills at earlier stages of their education as a matter of course.
That skill set includes principles of prompt engineering (prompting) as well as the understanding of different categories of and methods for identifying LLM hallucinations (a term often used to designate several types of errors in LLM output).
Finally, we conclude this presentation with real-world case studies of applying DI-AMP to three college-level assignments disrupted by the technology, and transform them into assignments that potentially produce similar learning outcomes and **deliberately, methodically, and reflexively** incorporate structured use of CG as a tool, not only to mitigate the negative effects of this disruptive technology, but also to help students develop a new set of skills for the new post-CG world.